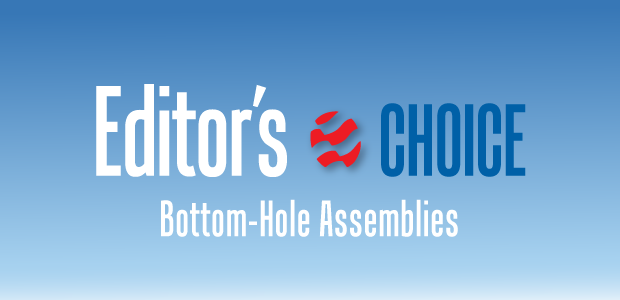
Data Unlocks More Useful Inspections
By Lonnie Smith
For years, the drilling industry has focused on optimizing bottom-hole assemblies to improve drilling performance. Since the last 90 feet of a drill string are its costliest components and the ones with the biggest financial impact, incremental changes to the BHA can help drillers consistently deliver high-quality wells at a low cost.
But reviewing BHA data to make adjustments is only one component of perfecting the drilling process. To maximize performance, operators and service companies will need to adopt comprehensive data collection that combines knowledge of the BHA with other practices, including quality control behaviors, continuous improvement initiatives and data science. The holistic data should be organized well enough that companies can track improvement trends and flag concerning indicators.
The easier it is for companies to leverage the data, the more valuable it will become. For data collection to be sustainable, the link between it and continuous improvement must be clear.
Figure 1 shows some of the information a drilling data ecosystem should contain. Since BHA components at the rig are owned by a complex web of tool manufacturers, getting such diverse, holistic data can be challenging, a reality that is particularly noticeable when an emergency occurs and data must be retrieved quickly. Fortunately, comprehensive data’s benefits more than justify the effort to collect it.
A Case In Point
Consider a Permian Basin operator that was having communication problems with a rotary steerable system. The RSS communicated using mud pulse telemetry, which sends information to the surface by restricting fluid flow to create pressure pulses. Such telemetry became unreliable as wear on the radial bearing allowed additional fluid to bypass the tool through the bearing assembly.
Communication issues could happen even though the motor bearing’s fit met the criteria for reuse established by the engineering team that designed the motor. Instead of looking at a specific application, these criteria attempt to accommodate all drilling conditions. Ideally, the standard fit allows some bypass to ensure drilling fluid can lubricate and cool the bearings during drilling, but remains tight enough to stabilize the bit box mandrel and endure loads from the power section as they transfer to the bit.
Motor bearings frequently are organized with two male and female bearing pairs configured as an upper and lower, with the lower bearing closest to the bit. Bearings usually are coated with a wear-resistant carbide on the outer diameter of the male bearing and the inner diameter of the female bearing.
When assembling motors, the tool manufacturer will mix and match the male and female pairs to ensure the bearing fit meets the design team’s all-purpose specification. Little to no consideration goes to the application in which the motor will be used. So long as the bearing meets the standard manufacturing tolerance, it is deemed suitable.
Quality Control
Quality control is essential to verify that mud motors and other downhole tools will perform as expected. As the industry seeks to solve BHA tool challenges with data, several quality control mechanisms need to be examined.
The first is the third-party nondestructive testing conducted between BHA tool trips to ensure equipment can run another time without failing. Today, the industry standard is for the company performing the nondestructive testing to be independent of the manufacturer. In theory, that encourages inspectors to evaluate the tool objectively and choose the most reliable equipment for the job.
Unfortunately, living up to that ideal can be challenging for even the most dedicated and conscientious inspectors. NDT companies work within the walls of tool manufacturers every day and naturally build strong relationships with those manufacturers rather than the operators they are supposed to look after but rarely see. Furthermore, the NDT company bills the tool manufacturer and relies on its patronage to remain in business.
These close ties undermine objectivity. Even without intentionally doing so, the tool manufacturer can exert extreme pressure on the NDT company to approve equipment that is questionable or should not be used at all.
Third-Party Monitors
To eliminate that pressure and improve BHA reliability, many operators have begun hiring third-party inspection services themselves. This approach requires the operator’s chosen third-party to be present during the entire process of preparing a tool to ship to the rig, including the tool’s selection, NDT inspection, repair and maintenance, and final logistics. While such third-party monitors may be unfamiliar with each tool, they are very well-versed in quality training and most American Petroleum Institute and DS-1® drilling tool guidelines.
Employing inspection services directly has two shortcomings. First, because the operators’ inspectors must be present, it adds significant costs to tool deployment, including both direct costs and indirect costs from slowing the process.
The other major drawback is how this service is measured as a success. There is a well understood feeling in the oil patch that an auditor who fails to find any issues is slacking off. As a result, many consider “number of findings” a major key performance indicator for inspectors. That works fine initially, but as quality improves, it has an unintended consequence: Eventually, the list of findings devolves into a chronicle of small, minor issues that are unlikely to impact drilling performance.
The TH Hill Manual for DS-1, which establishes and maintains many of the quality processes and criteria necessary when evaluating the drill string, sometimes reinforces the focus on inconsequential issues. While the manual leaves some leeway in how BHA tools should be evaluated, it largely is a one-size-fits-all standard. To ensure quality and uniformity across applications, it favors more thorough inspections even when the extra work can be superfluous.
Whether they are deployed in offshore or onshore environments, BHA components must perform in vastly different circumstances. The one-size-fits-all approach is not the best one for any drilling program, and it can have the side effect of increasing costs.
The standard also contains many gray areas with which the supply chain must wrestle. These gray areas can be a great source of consternation and do not necessarily lead to the most important outcome, which is a better tool down hole for the customer at the most economic rate.
Targeted Guidelines
Rather than relying on general standards, some operators develop their own quality guidelines to fine-tune requirements to a particular drilling program’s risks. Such customization is useful and should be in every operator’s toolkit, but it is very challenging to keep application-specific standards up-to-date, given how quickly downhole tool technology evolves. Custom standards also tend to explode in complexity as additional circumstances and drilling challenges become relevant.
Digital tools can empower operators to offer application-specific guidelines that keep pace with modern tools and remain user-friendly. To illustrate, let’s return to the Permian Basin operator who experienced RSS communication issues as bearing wear allowed more fluid through the motor’s bypass.
To run the motor of choice without those issues, the operator needed the ability to preselect the acceptable bearing fit based on expected motor conditions. In case the actual conditions deviated from the plan, the wear prediction process had to be simple enough to run repeatedly during drilling so the operator could see likely changes in bearing fit.
A simplistic prediction technique involves associating bearing wear with the number of hours the motor ran. Unfortunately, this approach is impractical, as how much wear the motor experiences during each hour varies based on the drilling conditions and a multitude of other factors. The variation is so high that suppliers would have to build in a large margin of error, leading to higher costs and inventory stress.
The operator can resolve the RSS communication issues by buying new bearings for each run, but that would have been expensive and wasteful even if the bearings had been available. Given the limited inventory, that approach was impossible. Instead, the operator sought a better way to predict wear.
Building a True Model
Machine learning offered a solution. With sufficient data, an ML algorithm can estimate bearing wear accurately enough to provide a useful inspection standard.
Fortunately, the operator had detailed data on its past runs. A select dataset was compiled into a matrix consisting of data related to the motor assembly’s pre-run condition, electronic data recorder, mud log, motor run, bit and post-run inspection. Information in these datasets included power section type, pre-run bearing measurements, motor bend degree, bearing coating, stabilization, amount of time the motor ran, motor depth, time rotating versus sliding, build angles while drilling, weight on bit, mud properties and post-run bearing measurements.
The dataset contained 468 unique instances of the preferred motor with all the other relevant features; 80% of the instances were randomly selected to train the model. The other 20% were set aside for validating the model’s ability to predict bearing fit after disassembly. After being fed all the pre-run, EDR and mud data, the model was expected to forecast bearing fit within a thousandth of an inch.
This dataset was fed into a stacked ensemble model, combining four base models. As Figure 2 notes, those models were:
- A gradient boosting machine;
- A distributed random forest;
- Deep learning; and
- A generalized linear model.
The meta-learner algorithm that is used to combine the base models’ predictions is an elastic-net regularized generalized linear model.
To assess and help prevent overfitting—the chance that the model would produce inaccurate predictions by giving too much weight to eccentricities in the training data—the model was evaluated using five folds in cross-validation. Overall, the stacked ensemble leveraged the complementary strengths of the base models, resulting in strong performance and achieving both high accuracy and generalization on the given dataset.
Accurate Results
The validation data indicates that the ML model used for predicting bearing fits performs very well, with low values for the root mean squared error, mean absolute error and root mean squared logarithmic error. The residual deviance and the null deviance values suggest that the model explains an overwhelming portion of the variance in the data. Table 1 presents the values for these metrics and a few others.
The model was trained to predict a value that had an average fit of .038 inches. The error rates are extremely small, with more than 50% of the error density within 5% of the actual measured size. This translates to more than half the predictions being within .0019 inches of the actual measured fit. All the error sample means are within 10% or .0038 inches of the actual measured fit (Figure 3).
Once the bearing fit predictor model was complete, the operator had a quick way to pre-qualify a bearing fit suitable for the expected drilling needs. The operator could supply an assumed number of hours, the motor configuration and the mud type to produce a bearing range that would prevent communication problems.
Additionally, the model could be fed real time EDR and mud data to estimate the current bearing fit, as well as produce a spline model forecasting what the bypass would be within a cone of certainty. These real-time updates enabled the operator to anticipate possible communication issues before they occurred and plan accordingly.
The existing quality plan then was updated with a framework to communicate the bearing tolerance window before the motor was built, ensuring the correct fit was obtained before additional motors arrived at the rig.
This solution was only possible because of groundwork laid ahead of time. Long before RSS communication issues arose, the operator committed to collecting, organizing and storing data. The specific dataset intentionally included both good runs and bad runs. This information was captured in a cloud-based system that could collect data from multiple tool manufacturing sites in real time, as well as from the rig while drilling.
Finally, the feedback loop of collecting data, reviewing that data to extract meaningful signals, and adjusting the quality plan was embraced by both the operator and its suppliers. This support enabled the learned solution to be instantly inserted into the supply chain flow with little disruption.
The mud motor supplier and other manufacturers appreciated the application-specific quality plans because they allowed subcomponents to be selected based on need. This saves money by freeing suppliers to draw from a broad pool rather than restricting themselves to new parts, regardless of the situation.
As machine learning tools continue to advance and collecting data gets easier, creating need-based quality standards will become increasingly common. It reduces unnecessary costs and risks for operators, gives suppliers flexibility that can translate into higher margins and faster delivery times, and helps independent inspectors concentrate on the most meaningful work.
But to take advantage of these emerging capabilities, operators and service companies must make collecting and retaining holistic data one of the foundational tenants of their operations. Fortunately, improving inspections is only one of many applications for the data. It will pay as-yet-unimagined dividends for decades to come.
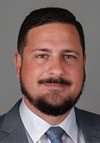
LONNIE SMITH is the president and co-founder of Turnco, a nondestructive testing specialist, and the inventor of the B.R.I.T.E. and D90 portal for providing real-time data on bottom-hole assembly tools’ performance. With more than 25 years of experience, Smith excels at solving BHA challenges for operators and drilling contractors alike. Before founding Turnco in 2010, he worked as a supply chain manager for Dyna-Drill Technologies, a Schlumberger subsidiary, and as a global asset manager for Pathfinder Energy Services. Smith holds an executive education certificate in energy from The University of Texas Red McCombs School of Business, as well as a B.A. in finance from the University of Houston.
For other great articles about exploration, drilling, completions and production, subscribe to The American Oil & Gas Reporter and bookmark www.aogr.com.