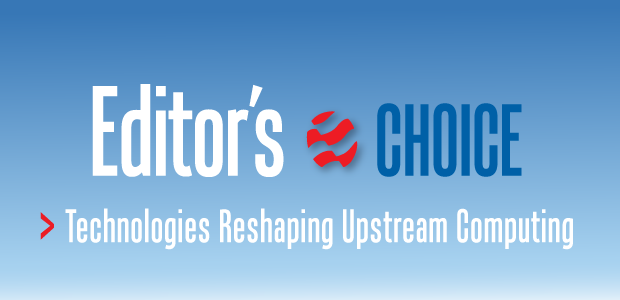
Synergies Between Analytics, Cloud And Pattern Recognition Reshaping Upstream Computing
By Murray Roth
LITTLETON, CO.–“SiriTM, what well spacing should I use in the Bakken?”
“I’m not sure what you said there.”
“Siri, what well spacing should I use in the Bakken?”
“What will spacing should I use in the Boston?”
“Siri, you’re no help at all.”
“You’re certainly entitled to that opinion.”
Okay, so maybe Siri is not quite ready to help operators make oil and gas decisions, which by nature, tend to be complex and rely on lots of data and technical inputs. But eventually, as I persisted, Siri did take me to websites describing hydraulic fracturing, proppant concentration and other industry specifics.
What roles will innovative consumer technologies such as Siri have in the oil and gas industry, and where are components of this technology being applied today?
Three main technologies are used whenever Siri is accessed from any phone in the world: pattern recognition, analytics and cloud computing resources. Based on information from a number of Internet sites, several coordinated steps were performed when I asked for Bakken well spacing operational advice.
First, my request was passed through a noise cancelling microphone before being encoded into digital format. These digital data are temporarily stored locally and also are transferred through an Internet connection to the Siri server on the cloud.
The cloud is the latest incarnation and name of an Internet service provision model where software and hardware are accessed as a service, with no need to either own or access this technology on a local computing device. The Siri server is loaded with artificial intelligence algorithms to interpret the digitized request and provide suitable feedback to the originating phone.
At the same time, locally on the phone, pattern recognition technology is used to determine the content of the request and whether the request can be resolved locally or requires access to Internet information or resources. On the server, the data are compared with various statistical algorithms to interpret the message and come up with a solution. A similar operation also is performed locally on the phone using a subset of the statistical algorithms, with Siri proceeding with the more probable of the two solutions. The accuracy of the final response is checked with a language model, and voice feedback is provided to the phone user. And all this occurs within a few seconds.
Before considering the impact that Siri, its equivalents and their extensions will have on the upstream oil and gas business, it is necessary to first consider how the complementary technologies of pattern recognition, analytic interpretation and modeling, and cloud computing access are being applied in the industry today.
Pattern Recognition
Rudimentary implementations of pattern recognition technology were first used to drive automated interpretation of seismic data more than 25 years ago. Similarity measurements of seismic data have made it possible to specify a limited number of control points for a geologic interface, and automated horizon trackers complete the interpretation. After sufficient editing or iterations, the result is a detailed structural map, useful for prospect identification, reservoir mapping, reservoir modeling and more.
Advances not only have increased the reliability of automated horizon interpretation, but also have streamlined the guided interpretation of geologic faults. Using image processing to identify the most fault-like features enables a modified voice pattern recognition algorithm to automatically track any selected fault feature (Figure 1). With a limited amount of guidance, these new algorithms can complete the definition of fault planes and dramatically boost the productivity of seismic interpretations.
FIGURE 1
Automatically Tracking Faults using
Pattern Recognition Algorithms
Modified voice recognition algorithms can be used to automatically track any selected vertical and horizontal fault features in processed seismic data. With a limited amount of guidance, these new algorithms can complete the definition of fault planes and dramatically boost the productivity of seismic interpretations.
Equivalent technology is being extended to other interpretive challenges, including the automation of geologic top picking, the digitizing of raster well logs into digital form, and seismic facies classification.
In the first case, unique well log and petrophysical characteristics can be classified around a geologist’s boundary interpretation. Moving to nearby well locations, the classified pattern can be searched for and identified to locate the equivalent geologic top. By allowing adaptive modification to the pattern classification, a single geologic interpretation can be extrapolated to hundreds or thousands of wells in a matter of seconds.
The tedious and error-prone task of digitizing well log images can be streamlined by ignoring the repetitive pattern of vertical and horizontal grid lines, and focusing on the image pattern of the desired well log information.
FIGURE 2
Classifying Seismic Facies Trends
By selecting a pattern analysis window, seismic waveform pattern classification can reveal subtle key reservoir features such as thickness, porosity or fluid content.
Finally, by selecting a pattern analysis window, unique seismic waveforms can be identified and used to classify seismic facies trends. These trends often are related to key reservoir features such as thickness, porosity or fluid content (Figure 2).
Envisioning beyond current innovations, pattern recognition technologies are equally applicable to production decline curve classifications, diagnostic injection responses, pressure and temperature anomalies, and more.
Applying Analytics
Analytics are routinely applied in financial markets, consumer marketing and professional sports, as popularized in the movie Moneyball, to sift through millions and billions of data samples to identify and act on meaningful trends. As a complement to pattern recognition, analytics can mathematically compare identified classifications with other data to model linear or nonlinear behavior.
For example, the New England Patriots football team analyzes game films to identify key defense patterns of opponents, and then uses analytics to determine which offensive plays will best exploit defensive weaknesses.
In the oil and gas industry, analytics are applied by engineers to model relationships among well production and other engineering parameters, such as horizontal well length, number of perforations, total fluid and proppant pumped, etc. The goal of this modeling is to identify what drives better well production, and thereby optimize the application of drilling and completions techniques. Increasingly, geologic parameters such as reservoir thickness, saturation, porosity and geochemical measurements of total organic carbon and thermal maturity are all being brought into the mix to better estimate what drives optimal well production.
FIGURE 3
Mapping Regional Variations in Geologic Prospectivity
Building reliable correlations among drilling and completions parameters and well production can help isolate the effect geologic conditions have on relative well performance. Analytic interpretation and modeling identify and account for engineering variability to map regional variations in the geologic prospectivity of possible well locations.
Building reliable correlations among key drilling and completions parameters and well production can help isolate the effect that geologic conditions have on relative well performance. Analytic interpretation and modeling identifies and accounts for engineering variability to map regional variations in the geologic prospectivity of possible well locations (Figure 3).
Analytic interpretation and modeling also are applied to geologic well logs to either predict missing well logs or well log sections, or to map petrophysical facies or lithology zones. In a similar fashion, geologic and geophysical maps or seismic volumes can be used to analytically model key facies trends.
Looking ahead, analytics are likely to play an ever-expanding role in the upstream industry, linking not only technical reservoir and engineering measurements, but also connecting drilling and completions costs, lifting costs and commodity pricing with field development optimization models.
Cloud Computing Access
Internet provision of computing hardware and software capabilities is not a new concept. In fact, Internet service provision technology swept through numerous industries more than 15 years ago. Access to financial and tax software through this model is among the best modern derivatives of this approach. Social media such as Facebook and LinkedIn are more recent incarnations of the cloud computing approach.
However, the oil and gas industry has been reticent to embrace the Internet provision of both software and hardware capabilities, although operators are increasingly deploying central computing services and data servers within their intranet environments to manage costs and provide information technology consistency.
The barriers for not fully embracing the cloud computing access model in the oil and gas industry have been related to data security, the volumes of data involved, uptime reliability concerns and overall performance issues. Until proven cost savings drive a reconciliation or reconsideration of these objections, major adoption of this technology is not anticipated.
However, the greatest opportunities for deploying cloud computing access may well be associated with real-time monitoring of field operations. As data are being streamed remotely from various field sensors, there are opportunities to collate and present the results through cloud computing software and hardware.
FIGURE 4
Microseismic Monitoring of Hydraulic Fracturing Operations
Microseismic data are monitored by software applications capable of accessing processed data through the Internet. A natural extension of this model is to host the software in a cloud computing model with hardware support, bringing cloud computing into the analytical and operational realms of microseismic monitoring.
For example, microseismic monitoring of hydraulic fracturing operations (Figure 4) or microseismic detection of casing failure or zone breakout are viable cloud computing candidates.
The Road Ahead
Pattern recognition, analytics and cloud computing are at various stages of deployment in the oil and gas industry. Pattern recognition has both the longest history and the most diverse application, often as a means to reliably automate interpretation activities in geophysics and geology.
Analytics most commonly are used by engineers, although recent deployments are incorporating geologic and geophysical data in an analytic interpretation and modeling approach, to better quantify and standardize reservoir characterization.
Cloud computing is the least mature of the technologies discussed and the one that has the greatest difficulty fitting industry models for security and reliability.
As Siri illustrates, there is a technical synergy between these technologies, and their influence and interfacing should be expected to expand dramatically in the oil and gas industry.
Consider a future scenario, perhaps in the Bakken/Three Forks play in North Dakota, where production and other engineering measurements are recorded and made accessible with a cloud computing model. Pattern recognition technology could look for unique characteristics of the oil production decline, water cut and other time-based measurements. With this backdrop of readily accessible data and identified patterns, analytic interpretation and modeling technology could infer key relationships and predictions.
For example, individual wells could be identified or even communicate when they are prime for a pumping unit (and help select a recommended size), maintenance operations, recompletions, and even enhanced recovery. As in other industries, user-friendly “dashboards” will provide practical tracking of analytic recommendations for engineers, and in this scenario, will monitor the status and implementation of operational decisions.
But what about Siri herself and future variations? As the analytic algorithms become more sophisticated and exposure to oil and gas terminology expands, this artificial intelligence technology is bound to become proficient at identifying sources of information and providing useful responses. Verbal requests for well details, connection to relevant technical information or identifying industry technical experts will become commonplace and more efficient than mouse-and-menu information access.
“Siri, I think you are going to become a useful aid for the oil and gas industry.” “I would like that.”
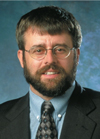
MURRAY ROTH is president and co-founder of Transform Software and Services Inc. in Littleton, Co. After graduating with an astrophysics degree from the University of Calgary, he began his career at Geophysical Service Inc., working in seismic acquisition, seismic processing and special project groups. Roth joined Landmark Graphics in 1989, contributing in roles spanning customer support, testing, product development and marketing. He served as executive vice president of research, development and marketing from 2002 until leaving in 2004 to form Transform Software and Services.
For other great articles about exploration, drilling, completions and production, subscribe to The American Oil & Gas Reporter and bookmark www.aogr.com.